In this study we consider the problem of how to design an optimal multichannel blind deconvolution (MBD) algorithm in the case where the probability density functions of the source signals are known.
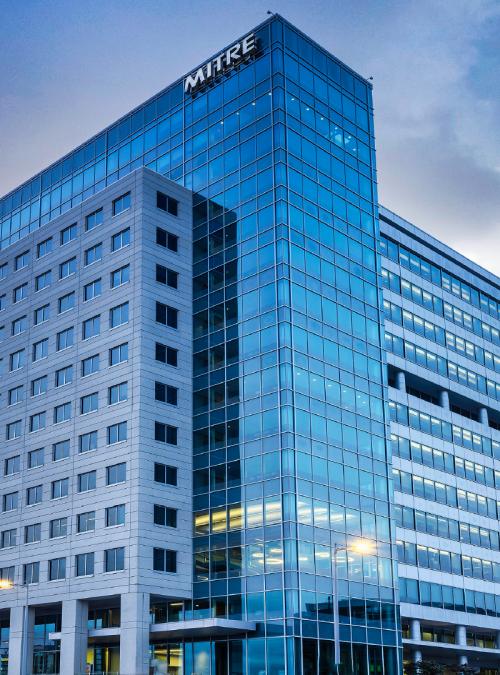
Optimal Multichannel Blind Deconvolution for Parameterized Channels and Known Source Densities (EDICS:SSP-SSEP)
Download Resources
PDF Accessibility
One or more of the PDF files on this page fall under E202.2 Legacy Exceptions and may not be completely accessible. You may request an accessible version of a PDF using the form on the Contact Us page.
In this study we consider the problem of how to design an optimal multichannel blind deconvolution (MBD) algorithm in the case where the probability density functions of the source signals are known. We assume existence of a parametric channel model that accurately characterizes the propagation environment. Through three major steps we derive a blind channel parameter estimator that is used to jointly compute the separation system and recover all the source signals. First, we replace the normally assumed nonparametric channel model with a physical model. Next, we introduce a symbolic pseudoinverse for our separation model to replace the ubiquitous inverse filter separation model. Thirdly, we introduce a minimum divergence estimator formulation to replace the commonly used minimum entropy formulation. We prove that the new estimator formed in this way is asymptotically consistent and Fisher-efficient. Through simulation we show the superior performance of our algorithm compared with existing techniques based on entropy minimization and inverse filter separation.