While a review of published work may suggest that the negation detection task in clinical NLP has been “solved,” our analysis of negation detection indicates it is easy to optimize for a single corpus but not to generalize to arbitrary clinical text.
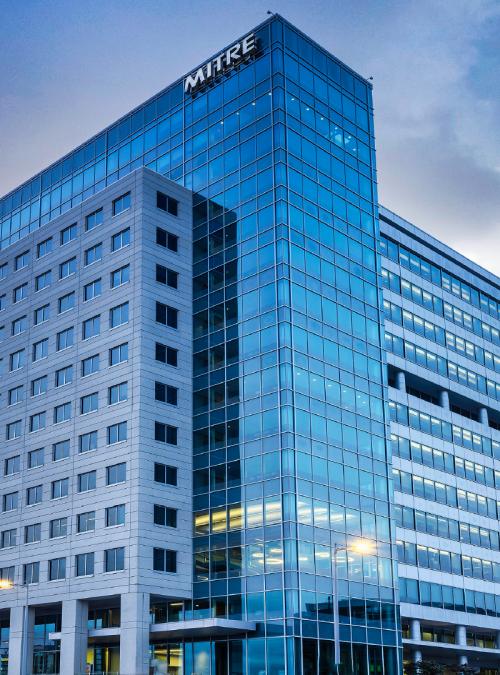
Negation's Not Solved: Generalizability versus Optimizability in Clinical Natural Language
Download Resources
PDF Accessibility
One or more of the PDF files on this page fall under E202.2 Legacy Exceptions and may not be completely accessible. You may request an accessible version of a PDF using the form on the Contact Us page.
A review of published work in clinical natural language processing (NLP) may suggest that the negation detection task has been "solved." This work contends that an optimizable solution does not equal a generalizable solution. Using four manually annotated corpora of clinical text, we show that negation detection can be optimized in relatively constrained settings, but performance is not reliably generalizable unless in-domain training data is available – in which case fullysupervised domain adaptation techniques may prove effective. Various factors (e.g., annotation guidelines, named entity characteristics, the amount of data, and lexical and syntactic context) play a role in making generalizability difficult, but none completely explains the phenomenon. This indicates the need for future work in domain-adaptive and task-adaptive methods for clinical NLP.