This keynote addresses current and future challenges in terminology and knowledge engineering focusing on multidocument, multilingual and multimodal information extraction.
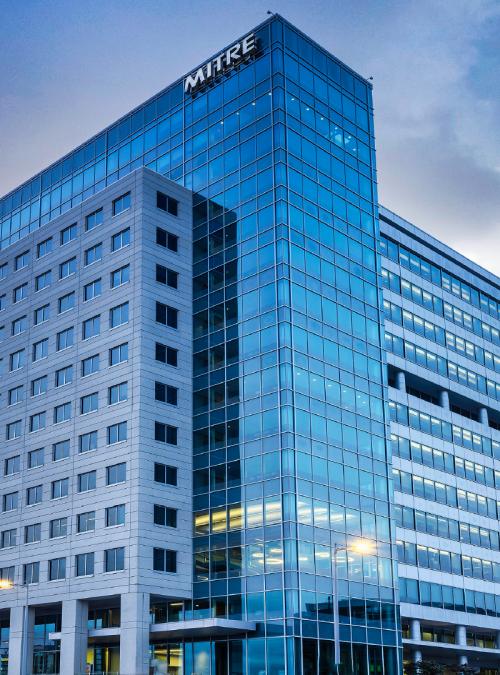
Multidocument, Multilingual, and Multimodal Information Extraction for Real World Applications
Download Resources
PDF Accessibility
One or more of the PDF files on this page fall under E202.2 Legacy Exceptions and may not be completely accessible. You may request an accessible version of a PDF using the form on the Contact Us page.
This keynote addresses current and future challenges in terminology and knowledge engineering focusing on multidocument, multilingual and multimodal information extraction. With some reports that humanity creates more than an exabyte (1018 bytes) of unique information each year, the imperative for tools to mitigate the size, heterogeneity, and complexity of knowledge collections is a priority. After exemplifying this grand challenge in typical real world analytic environments, we briefly review the state of the art in information access. We note that automated systems exist that can return documents relevant to a particular subject with around 80% precision but low recall. Automated document query incorporating relevance feedback has achieved near human performance. Extraction of named entities (Hirschman 1998) is over 90% accurate and extraction of relations among entities in specific domains is about 70-80% accurate. Also, documents can be summarized to about 20% of their source size without information loss, which can save users 50% of their original task time. Finally, prototype systems can respond to a simple factual question by returning answers from relevant documents with about 75% accuracy.