Emergence of new infectious agents spreading at a global scale has added new urgency to the development of real-time disease surveillance systems.
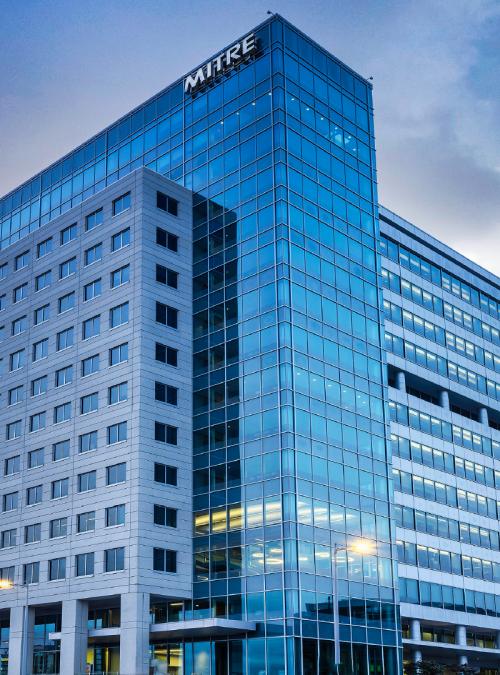
A Family of Temporal Surveillance Techniques for Detecting Increasing Outbreaks
Download Resources
PDF Accessibility
One or more of the PDF files on this page fall under E202.2 Legacy Exceptions and may not be completely accessible. You may request an accessible version of a PDF using the form on the Contact Us page.
Emergence of new infectious agents spreading at a global scale has added new urgency to the development of real-time disease surveillance systems. At the same time, biological terrorism is a growing concern. The successful interdiction against SARS demonstrated the confrontation of these urgent crises through rapid and accurate detection of unusual epidemiological trends. Many surveillance algorithms have been proposed in the literature, but comparing these approaches is complicated. Benchmarking of temporal surveillance techniques is a critical step in the development of an effective syndromic surveillance system. Unfortunately, holding "bake-offs" to blindly compare approaches is a difficult and often fruitless enterprise, in part due to the parameters left to the final user for tuning. In this paper, we demonstrate how common analytical development and analysis may be coupled with realistic data sets to provide insight and robustness when selecting a surveillance technique. Four detection approaches, all part of a family of detectors, are considered: G-surveillance based on the space-time scan statistic, a uniformly most powerful test for exponentially increasing outbreaks, a monotonic regression approach, and a non-negative regression approach. The first of the four is a standard technique, while the latter three are new techniques for syndromic surveillance. All are compared using time series on patient visits coded as influenza-like illness at Harvard Pilgrim Health Care in the Boston area. The tradeoff in detection capability and robustness demonstrates the benefit of a monotonic regression approach for growing outbreaks. If an exponentially growing outbreak is the target, then the uniformly most powerful test delivers nearly optimal performance.