This study's goal was to model airspace Dynamic Density and complexity (and hence controller workload) using traffic characteristic metrics.
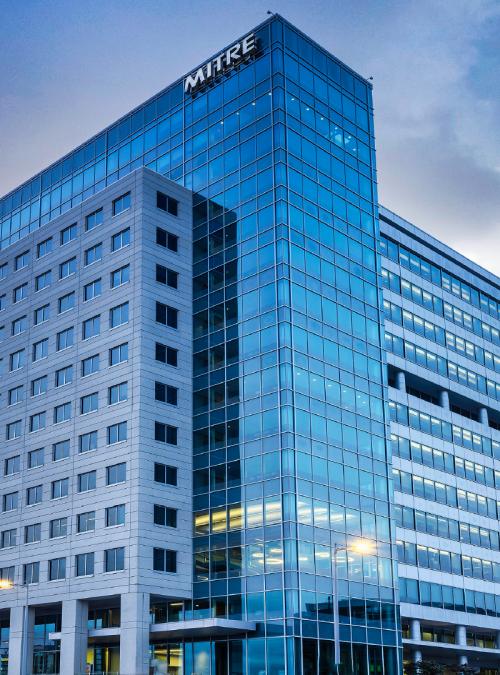
Dynamic Density and Complexity Metrics for Realtime Traffic Flow Management
Download Resources
PDF Accessibility
One or more of the PDF files on this page fall under E202.2 Legacy Exceptions and may not be completely accessible. You may request an accessible version of a PDF using the form on the Contact Us page.
This study's goal was to model airspace Dynamic Density and complexity (and hence controller workload) using traffic characteristic metrics. The focus was on metrics that could eventually enable Traffic Flow Management (TFM) personnel to strategically prevent overloads using triggers other than predicted sector traffic count. Potential metrics from past studies were assessed in terms of how well they could be predicted at time horizons required for TFM decision support (up to 120 minutes), and their face validity. Also, proportional odds logistic regression determined the metrics' usefulness for predicting subjective complexity ratings collected in an FAA-NASA study. Based on these analyses, a subset of 12 metrics was chosen (from the original 41). Further multiple regression analyses were conducted with this reduced model, to determine which metrics provided unique contributions to the prediction of subjective complexity, and to see the extent to which the same complexity factors related to subjective workload in different airspaces. Structured interviews with a sample of eight Traffic Management Coordinators were used to cross-check the quantitative findings. Specific aircraft proximity, density, and airspace structure metrics were found potentially useful for real-time TFM decision support. Many of the useful metrics were normalized and smoothed measures from an algorithm developed by Wyndemere, Inc. Also, it was found that different metrics related to subjective complexity in different centers, but the differences were small enough that a generalized set of complexity metrics might be applicable to multiple airspaces, at least in the near term. Future work could determine the viability of airspace-adapted complexity algorithms. The fact that multiple types of metrics are useful suggests that a multidimensional visual representation of predicted workload might be useful in TFM, as opposed to combining all relevant factors into a single metric.