Previous work has shown the potential advantages of using endogenous fitness schemes in classifier systems.
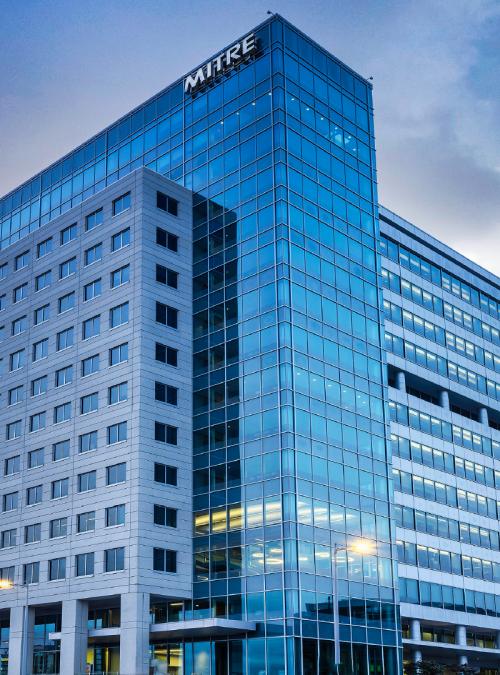
Classifier Systems, Endogenous Fitness, and Delayed Rewards: A Preliminary Investigation
Download Resources
PDF Accessibility
One or more of the PDF files on this page fall under E202.2 Legacy Exceptions and may not be completely accessible. You may request an accessible version of a PDF using the form on the Contact Us page.
Previous work has shown the potential advantages of using endogenous fitness schemes in classifier systems. The basic idea behind endogenous fitness is to reinforce successful system performance with "resources" that rules need in order to reproduce. Instead of storing explicit quantitative estimates of performance, each rule has one or more reservoirs that are used to store resources. When enough resources have been accumulated, a rule utilizes some of its resources to reproduce and the reservoir level is reduced accordingly. This paper extends this concept to accommodate environments having delayed rewards. Reinforcement learning techniques for solving average-reward Markovian decision processes are combined with a simple endogenous fitness scheme in a classifier system. We describe initial tests of this approach on state space search problems used in previous classifier system studies.