The threat of biological warfare and the emergence of new infectious agents spreading at a global scale have highlighted the need for major enhancements to the public health infrastructure.
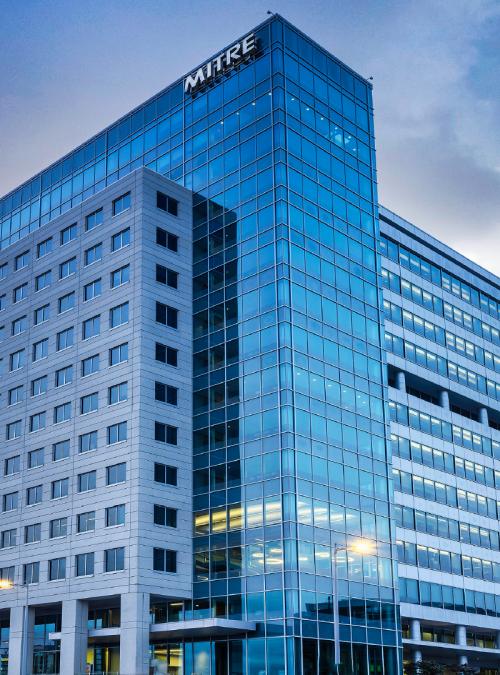
A Binomial Model of Transients in Daily ED Visits for Detecting Infectious Disease Outbreaks
Download Resources
PDF Accessibility
One or more of the PDF files on this page fall under E202.2 Legacy Exceptions and may not be completely accessible. You may request an accessible version of a PDF using the form on the Contact Us page.
The threat of biological warfare and the emergence of new infectious agents spreading at a global scale have highlighted the need for major enhancements to the public health infrastructure. Effective confrontation of these urgent crises requires rapid and accurate detection of unusual epidemiologic trends, for which our current surveillance capabilities are not adequate. Critical for real time surveillance are two components: real-time data and real-time interpretation of data. Today, most existing surveillance systems are capable of monitoring and capturing real time data. However, the state of practice for detecting temporal and spatial abnormalities in surveillance data remains inadequate. We introduce a locally stationary binomial model of early detection of epidemiologic events, applied to real historical data pertaining to the daily number of visits with respiratory syndromes to the emergency department (ED). We show that when simulated outbreaks are introduced into the respiratory data, our uniformly most powerful detection algorithm under a constant false alarm rate is capable of detecting such irregularities in the data with high sensitivity, specificity, and in a timely manner.