In this review, we summarize recent accomplishments in literature data mining for biology.
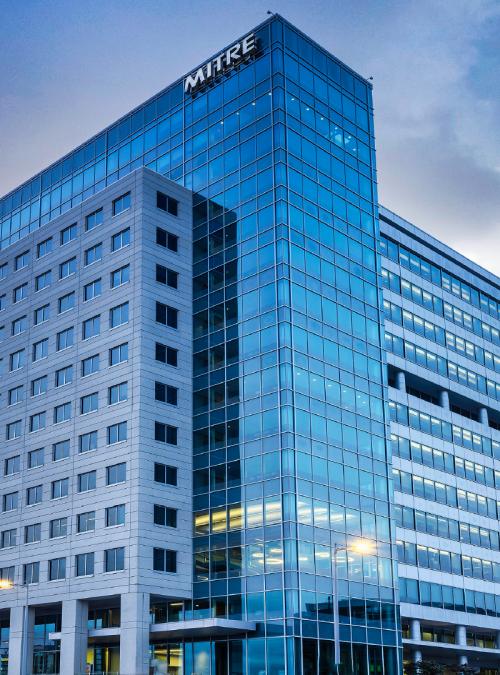
Accomplishments and Challenges in Literature Data Mining for Biology
Download Resources
PDF Accessibility
One or more of the PDF files on this page fall under E202.2 Legacy Exceptions and may not be completely accessible. You may request an accessible version of a PDF using the form on the Contact Us page.
In this review, we summarize recent accomplishments in literature data mining for biology. We then discuss the need for a challenge evaluation for this field, and initial steps to create such an evaluation. Literature data mining has progressed from simple recognition of terms to extraction of interaction relationships from complex sentences, and has broadened from recognition of protein interactions to a range of problems such as improving homology search, identifying cellular location, or recognizing trends and themes in the literature. Given this explosion of research, we argue that the time is now right to create a challenge evaluation focused on one or more problems of immediate biological relevance. A challenge evaluation will give rise to a shared infrastructure, including annotated training and test data, and shared evaluation methods. This would enable researchers to compare approaches and share information, leading to accelerated progress in the field. In this context, we describe two specific applications: extraction of biological pathways from the literature and automated database curation. For each of these, we outline the task definition, the creation of an annotated corpus, and evaluation metrics.