We describe an experiment to elicit judgments on the validity of gene-mutation relations in MEDLINE abstracts via crowdsourcing.
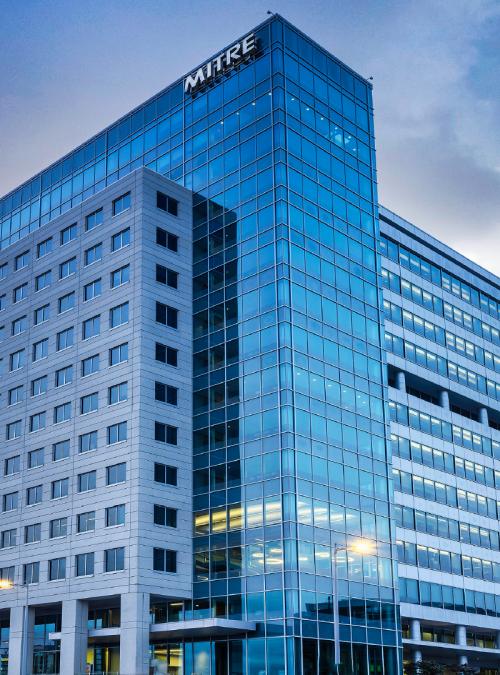
Validating Candidate Gene-Mutation Relations in MEDLINE Abstracts via Crowdsourcing
Download Resources
PDF Accessibility
One or more of the PDF files on this page fall under E202.2 Legacy Exceptions and may not be completely accessible. You may request an accessible version of a PDF using the form on the Contact Us page.
We describe an experiment to elicit judgments on the validity of gene-mutation relations in MEDLINE abstracts via crowdsourcing. The biomedical literature contains rich information on such relations, but the correct pairings are difficult to extract automatically because a single abstract may mention multiple genes and mutations. We ran an experiment presenting candidate gene-mutation relations as Amazon Mechanical Turk "HITs" (human intelligence tasks). We extracted candidate mutations from a corpus of 250 MEDLINE abstracts using EMU combined with curated gene lists from NCBI . The resulting document-level annotations were "projected" into the abstract text to highlight mentions of genes and mutations. Turkers returned results within 30 hours. We evaluated the aggregated weighted results against a gold standard of expert curated gene-mutation relations. Weighted accuracy was 82%, with the best Turker achieving over 95% accuracy. The experiment demonstrates feasibility of attracting proficient annotators and the success of the interface in facilitating these judgments.