To characterize and ameliorate the weaknesses of clinical negation detection techniques across corpora that have different annotation schema.
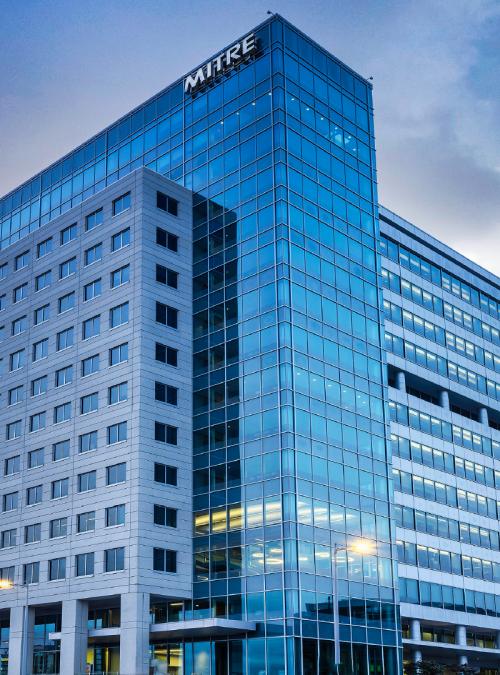
Negation's Not Solved: Reconsidering Negation Annotation and Evaluation
Download Resources
PDF Accessibility
One or more of the PDF files on this page fall under E202.2 Legacy Exceptions and may not be completely accessible. You may request an accessible version of a PDF using the form on the Contact Us page.
Though negation detection is a straightforward task in relatively constrained settings, when evaluated in heterogeneous corpora and annotation schema, performance may fall well below that of published benchmarks for both machine learning-based and rule-based approaches. Furthermore, it is difficult to determine the optimal mix of training data, or a standardized way to constrain evaluation metrics, since both are influenced by the corpus and annotation characteristics.