Data quality, defined here as fitness for use, is increasingly seen as a serious problem in government and private sector databases.
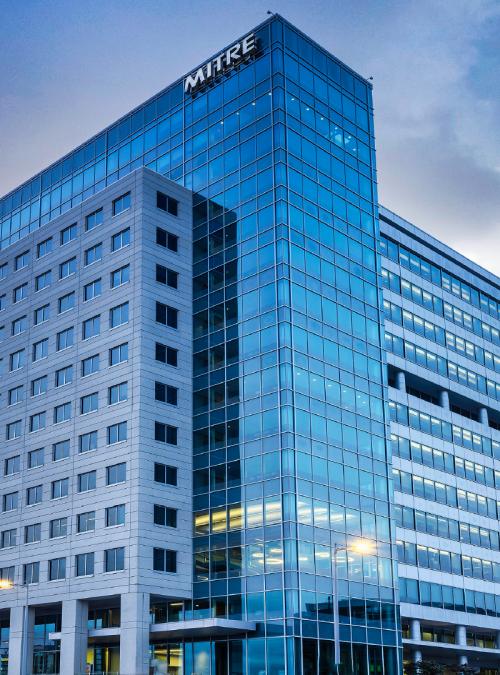
Methodology for Intelligence Database Data Quality
Download Resources
PDF Accessibility
One or more of the PDF files on this page fall under E202.2 Legacy Exceptions and may not be completely accessible. You may request an accessible version of a PDF using the form on the Contact Us page.
Data quality, defined here as fitness for use, is increasingly seen as a serious problem in government and private sector databases. We will survey available techniques, and then describe our own work. We are adapting general data quality techniques suited to intelligence databases, focusing on an aspect rarely seen in the literature, i.e., helping an intelligence analyst assess individual data records/objects. Our emphasis is on developing solutions to the problem of providing better consumer information on each value used. We provide such information, so the consumer can determine whether the data are good enough for the intended purpose. The primary concern is with individual data items that drive major decisions, where erroneous data have high cost (e.g., human lives). The broad aim is to enable better decisions. A narrower aim is for consumers to trust data when appropriate, thereby reducing the incentives to ignore the data or expend effort on workarounds for data of unknown quality. This paper explains where our approach fits in the spectrum of data quality approaches, and describes a methodology for providing intelligence analysts (consumers) with information needed to guide how they use each data value in making decisions. The methodology encompasses the following aspects: Providing an infrastructure to define, store, and make available quality attributes on various data records/objects; Obtaining values for quality attributes on important data granules; Making the quality attribute values available to users of each data granule (including both humans and queries); Tracking the impact of providing the quality values, on decision makers and decisions.