Authorship analysis is a field of study which aims to infer authorship information from a document or group of documents. This paper develops an approach, BayesAD, for solving the authorship decomposition problem when there are multiple authors involved.
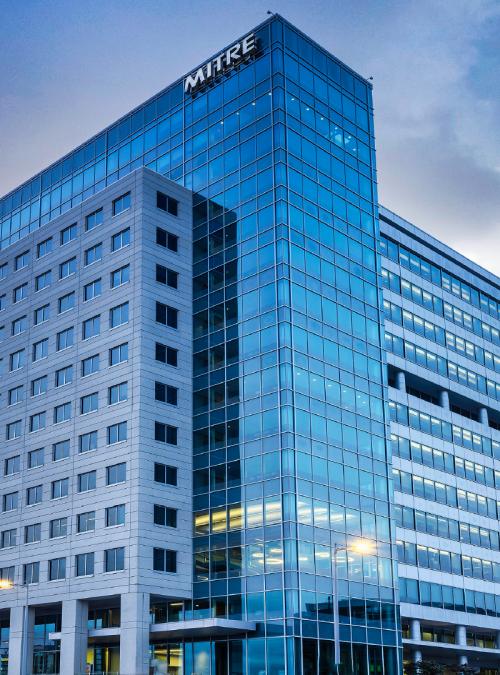
An Improved Algorithm for Unsupervised Decomposition of a Multi-Author Document
Download Resources
PDF Accessibility
One or more of the PDF files on this page fall under E202.2 Legacy Exceptions and may not be completely accessible. You may request an accessible version of a PDF using the form on the Contact Us page.
This paper addresses the problem of unsupervised decomposition of a multi-author text document: identifying the sentences that were written by each author assuming the number of authors is unknown. An approach, BayesAD, is developed for solving this problem: apply a Bayesian segmentation algorithm, followed by a segment-clustering algorithm. Results are presented from an empirical comparison between BayesAD and AK, a modified version of an approach published by Akiva and Koppel in 2013.
BayesAD exhibited greater accuracy than AK in all experiments. However, BayesAD has a parameter that needs to be set and which had a non-trivial impact on accuracy. Developing an effective method for eliminating this need would be a fruitful direction for future work. When controlling for topic, the accuracy of BayesAD and AK were, in all but one case, worse than a baseline approach wherein one author was assumed to write all sentences in the input text document. Hence, room for improved solutions exists.