The most significant open challenge to the worm defense community is to develop a sensitive detection method that can detect new worms in real time with a tolerable false alarm rate.
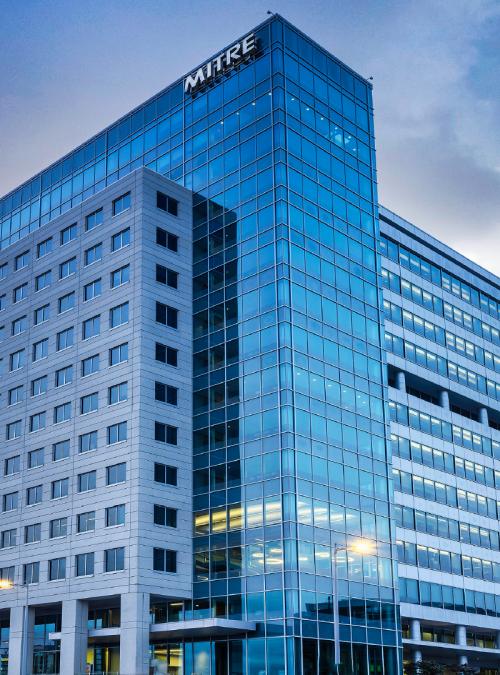
Graph-based Worm Detection On Operational Enterprise Networks
Download Resources
PDF Accessibility
One or more of the PDF files on this page fall under E202.2 Legacy Exceptions and may not be completely accessible. You may request an accessible version of a PDF using the form on the Contact Us page.
The most significant open challenge to the worm defense community is to develop a sensitive detection method that can detect new worms in real time with a tolerable false alarm rate. This paper presents a graph-based detection system and validates it on operational enterprise network data. We argue that the result is significantly closer to solving this challenge than other published works. We show that a graph-based approach to worm detection in an enterprise network can detect a broad range of active worms with a false alarm rate of less than twice per day. The supporting analysis comes from running the detection algorithm on a real enterprise network. The sensitivity results are significantly better than what is reported in the literature. We can detect all active, fast-spreading unimodal worms, including hit-list, topological, subnet-scanning, and meta-server worms.