The inherent dimension of hyperspectral data is commonly estimated for the purpose of dimension reduction.
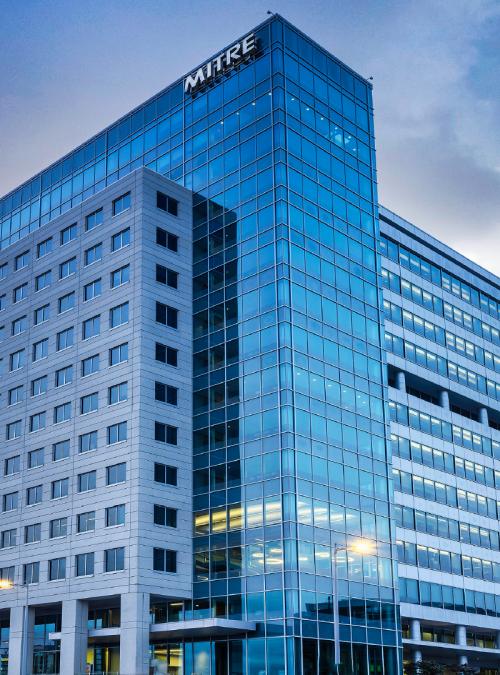
A Comparison Study of Dimension Estimation Algorithms
Download Resources
PDF Accessibility
One or more of the PDF files on this page fall under E202.2 Legacy Exceptions and may not be completely accessible. You may request an accessible version of a PDF using the form on the Contact Us page.
The inherent dimension of hyperspectral data is commonly estimated for the purpose of dimension reduction. However, the dimension estimate itself may be a useful measure for extracting information about hyperspectral data, including scene content, complexity, and clutter. There are many ways to estimate the inherent dimension of data, each measuring the data in a different way. This paper compares a group of dimension estimation metrics on a variety of data, both full scene and individual material regions, to determine the relationship between the different estimates and what features each method is measuring when applied to complex data.