Transformation-based learning has been successfully employed to solve many natural language processing problems. It has many positive features, but one drawback is that it does not provide estimates of class membership probabilities. In this paper, we present a novel method for obtaining class membership probabilities from a transformation-based rule list classifier. Three experiments are presented which measure the modeling accuracy and cross-entropy of the probabilistic classifier on unseen data and the degree to which the output probabilities from the classifier can be used to estimate confidences in its classification decisions. The results of these experiments show that, for the task of text chunking*, the estimates produced by this technique are more informative than those generated by a state-of-the-art decision tree. *All the experiments are performed on text chunking. The technique presented is general-purpose, however, and can be applied to many tasks for which transformation-based learning performs well, without changing the internals of the learner.
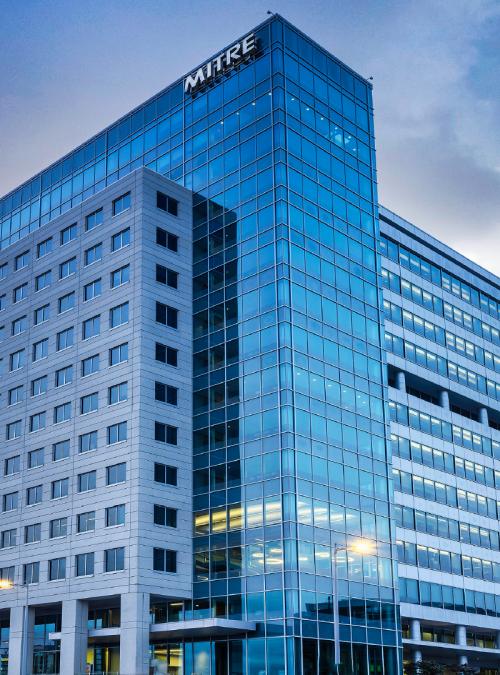
Coaxing Confidences From an Old Friend: Probabilistic Classifications From Transformation Rule List
Download Resources
PDF Accessibility
One or more of the PDF files on this page fall under E202.2 Legacy Exceptions and may not be completely accessible. You may request an accessible version of a PDF using the form on the Contact Us page.