Text annotation is an expensive pre-requisite for applying data-driven natural language processing techniques to new datasets. Tools that can reliably reduce the time and money required to construct an annotated corpus would be of immediate value to MITRE's sponsors. To this end, we have explored the possibility of using active learning strategies to aid human annotators in performing a basic named entity annotation task. Our experiments consider example-based active learning algorithms that are widely believed to reduce the number of examples and therefore reduce cost, but instead show that once the true costs of human annotation is taken into consideration the savings from using active learning vanishes.
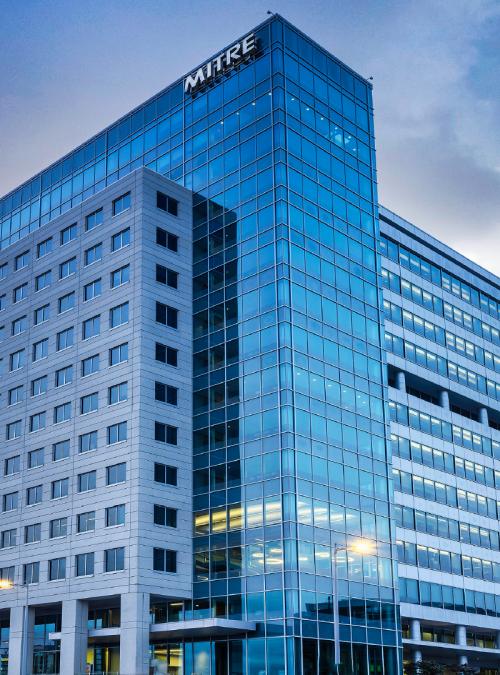
Active Learning with a Human In The Loop
Download Resources
PDF Accessibility
One or more of the PDF files on this page fall under E202.2 Legacy Exceptions and may not be completely accessible. You may request an accessible version of a PDF using the form on the Contact Us page.